Keynote Speakers of
ICCBB 2024

In order to deepen the communication in all the participants, ICCBB 2025 have invited
professors from all over the world to have speeches about
Computational Biology and Bioinformatics and related fields.

Keynote Speaker I
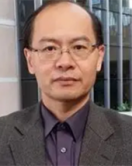
Prof. Luonan Chen
Distinguished Adjunct Professor, H-index: 81
Chinese Academy of Sciences/Hangzhou Institute for Advanced Study, UCAS,
China
Luonan Chen received BS degree in the Electrical Engineering from
Huazhong University of Science and Technology, and the M.E. and Ph.D.
degrees in the electrical engineering from Tohoku University, Sendai,
Japan, respectively. From 1997, he was an associate professor of the
Osaka Sangyo University, Osaka, Japan, and then a full Professor. Since
2010, he has been a professor and executive director at Key Laboratory
of Systems Biology, Shanghai Institute of Biochemistry and Cell Biology,
Chinese Academy of Sciences; Chair Professor of Hangzhou Institute for
Advanced Study, University of Chinese Academy of Sciences. He was
elected as the founding president of Computational Systems Biology
Society of OR China, and Chair of Technical Committee of Systems Biology
at IEEE SMC Society. In recent years, he published over 400 journal
papers and four monographs (books) in the area of bioinformatics,
nonlinear dynamics and machine learning.
Speech Title: "Dynamical
Data Science and AI for Biology and Medicine"
Abstract: I will present our recent
works on "Dynamical Data-Science and AI Applications" , including
dynamic network biomarkers (DNB) for early-warning signals of critical
transitions, spatial-temporal information (STI) transformation for
short-term time-series prediction, partial cross-mapping (PCM) for
causal inference among variables, and further AI4Science and Biology4AI.
These methods are all data-driven or model-free AI approaches but based
on the theoretical frameworks of nonlinear dynamics. We show the
principles and advantages of dynamical data-driven approaches with AI
for phenotype quantification as explicable, quantifiable, and
generalizable. The dynamical data-science and optimization approaches
with AI for the quantifications of phenotypes will further play an
important role in the systematical research of various fields in biology
and medicine.
Keynote
Speaker II
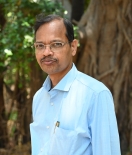
Prof. M. Michael Gromiha
H-index: 63
Indian Institute of Technology Madras, India
M Michael Gromiha is working as a Professor at the Department of
Biotechnology, Indian In-stitute of Technology (IIT) Madras, India. His
main research interests include protein structure and function,
mutational effects and development of bioinformatics databases and
tools. He has published more than 270 research articles, 70
reviews, eight editorials, and three books entitled “Protein
Bioinformatics”, “Protein Interactions” and “Protein mutations”. His
papers received more than 15,500 citations and his h-index is 63. He has
guided 23 PhD students, 10 Post-docs and handled more than 20 national
and international projects. He has organized five international
confer-ences, chaired scientific sessions and delivered
keynote/plenary/invited lectures in more than 200 na-tional and
international meetings. He is an Associate Editor of BMC Bioinformatics,
Frontiers in Bio-informatics and Bioinformatics Advances, Section Editor
of Current Protein and Peptide Science as well as an Editorial board
member of Scientific Reports, Biology Direct, Journal of Bioinformatics
and Computational Biology, Genes and Current Computer Aided Drug Design.
He has received several awards including the Oxford University Press
Bioinformatics prize, Okawa Science Foundation Research Grant, Young
Scientist Travel awards from ISCB, JSPS, AMBO and ICTP, Best Paper Award
at ICIC2011, ICTP Associateship Award, ICMR International Fellowship for
Senior Biomedical Scientists, INSA Senior Scientist Award, Best Paper
Award in Bioinformatics by the Department of Biotechnology, India,
Institute Research and Development Award from IIT Madras, Outstanding
Performance Award from Initiative for Parallel Bioinformatics (IPAB),
Tokyo Institute of Technology, Japan, Tamilnadu Scientist Award (TANSA)
from Tamilnadu State Council for Science and Technology, India and ASC
Masila Vijaya Award for excellence in research and pubi-cation from
Chennai Academy of Sciences. He is ranked as one of the topmost 0.5% of
highly cited re-searchers in the world and No.1 Scientist in
Bioinformatics in India in 2020. He is an elected Fellow of Indian
National Science Academy (FNA) and Academy of Sciences, Chennai.
Speech Title: "Development
of Databases and Computational tools for Understanding and Predicting
the Binding Affinities of Protein Complexes"
Abstract: The interactions of
proteins with other molecules are important for several biological
functions (1). The strength of these interactions is accounted with
binding affinity. Further, mutation of amino acids in the interfaces of
protein complexes alter their structure, binding affinity and function,
and some of them lead to diseases (2). The binding affinities of protein
complexes are related with sequence and structural features of residues
at the interface. We have developed thermodynamic databases for
protein-protein (3), protein-nucleic acid (4) and protein-carbohydrate
databases (5) and derived several parameters including contact
potentials, interaction energies and solvent accessibility to relate
with binding affinity. Based on the insights gained from this analysis,
we have developed computational tools for predicting the binding
affinity of protein-protein (6), protein-DNA, protein-RNA (7) and
protein-carbohydrate complexes. Further, we have elucidated the features
important for the binding affinity change upon mutation and developed
algorithms for predicting the change in binding affinity (8,9). These
resources help to relate the binding affinity with disease-causing
mutations, and designing therapeutic strategies for diseases (10).
Keynote
Speaker III
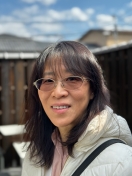
Prof. Hsueh-Fen Juan
H-index: 49
National Taiwan University, Taiwan
Dr. Hsueh-Fen Juan is a Distinguished
Professor in the Department of Life Science and the Graduate Institute
of Biomedical Electronics and Bioinformatics at National Taiwan
University. She serves as the Deputy Vice President for Research &
Development and the Director of the Center for Computational and Systems
Biology at the same institution. Dr. Juan is a pioneering systems
biologist and co-founder of the Center for Systems Biology at the
National Taiwan University. Her seminal research integrates multiple
omics techniques with bioinformatics to study human diseases and develop
novel drug discovery approaches. Her innovative methods have
significantly advanced cancer research, particularly in elucidating
molecular mechanisms of drug responses in cancer cells. A standout
achievement is her work on targeting ectopic ATP synthase for cancer
therapy, which garnered international attention in Science Daily and the
American Chemical Society's weekly news. Dr. Juan's contributions have
been recognized with numerous prestigious awards, including the 2008
Taiwan Ten Outstanding Young Person award, the 2012 K. T. Li
Breakthrough Award, and the 2019 MOST Outstanding Research Award. Her
research impact is evident in her impressive publication record: 155
journal papers, over 210 invited talks worldwide, and an h-index of 50
with more than 20,000 citations.
Speech Title: "Single-Cell Omics:
Revolutionizing Precision Medicine for Predicting Drug Response"
Abstract: The true potential of precision
medicine lies in its ability to tailor treatments to individual patients
based on their unique genetic profiles. Predicting drug responses
through individual transcriptomic profiles offers immense promise for
refining prognoses and advancing personalized treatments. While many
studies have focused on predicting responses of known drugs to new
transcriptomic profiles, research into responses for newly discovered
drugs remains limited. Single-cell omics is transforming this landscape
by enhancing our ability to predict drug responses and understand immune
cell dynamics in cancer. In this presentation, I will introduce scDrug+,
an innovative pipeline that seamlessly integrates single-cell RNA
sequencing (scRNA-seq) analysis with drug-response prediction. Unlike
existing methods that target known drugs, scDrug+ can predict responses
to new drugs by analyzing their molecular structures. This powerful tool
not only streamlines the drug repurposing process but also advances
prediction capabilities for novel therapeutics. Additionally, I will
discuss how single-cell omics advancements are shaping precision
medicine for drug prediction in T cell dynamics, cancer treatment, and
addressing long COVID. This presentation will provide insights into the
future directions of personalized medicine with the help of scDrug+ and
single-cell omics.
Invited Speakers

Invited Speaker I
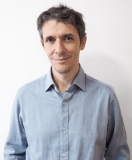
Assoc. Prof. Enrico Marsili
H-index: 39
University of Nottingham Ningbo China, China
Dr Enrico Marsili received his doctorate
in Chemical Engineering from the University of Rome, Italy. After
postdoctoral research at University of Minnesota, he took a Lecturer
position at Dublin City University. In 2012, he joined the newly formed
Singapore Centre for Environmental Life Sciences Engineering, Singapore,
as Principal Scientist. In 2019, he moved to Nazarbayev University as
Associate Professor in the Department of Chemical and Materials
Engineering. Since 2022, he is Associate Professor in Life Sciences and
Healthcare at University of Nottingham, Ningbo, China. His research
focuses on the characterization of mixed biofilms using electrochemical
methods and the development of novel bioprocess for efficient
biosynthesis of commodity chemicals in biofilms. He collaborates with
several institutions in China, such as South China University of
Technology (SCUT), Shenzhen Institute of Advanced Technology (SIAT) and
Shanghai Institute of Materia Medica (SIMM). He has received competitive
funding from Collaborative Research Programme and Marine Research
Program (National Research Foundation, Singapore), Public Utility Board,
Singapore, and municipal level funding (Ningbo, China). Dr Marsili has
published key contributions in Biofilm Electrochemistry and weak
electricigens on PNAS, Electrochimica Acta, and Bioresource Technology.
To date, he has published 83 papers, which have received over 7900
citations.
Speech Title: "Biofilm
Electrochemistry: from Characterization to Applications"
Abstract: Biofilms comprise of
microorganisms encased in self-produced extracellular polymeric matrix,
which provide mechanical stability, resistance to antimicrobials, and
favors adhesion to nearly any surfaces. When biofilms grow onto
electrodes, they are termed electroactive biofilm (EABs). EABs are
capable of extracellular electron transfer (EET) to and from solid
acceptor, through direct or mediated mechanism. EABs are beneficial to
wastewater treatment and could find applications in advanced
bioprocesses. A thorough comprehension of the mechanism underlying EET
is needed for biofilm management and to develop productive EABs for
bioprocesses, biomedical, and biosensing applications. The EET
mechanisms are investigated through a combination of electrochemical
techniques, molecular biology and microscopy techniques. Following early
studies on strong electricigens like Geobacter sp. and Shewanella sp.,
recent research has shown that most prokaryotes and even few eukaryotes
can be classified as EAB under specific conditions, thus extending the
validity of electrochemical methods for biofilm analysis. Further, EET
in weak electricigens is advantageous to design novel bioprocess like
electrofermentation, in which biopolymers in biofilms are produced at
higher yield or different properties than conventional fermentation
processes. In this presentation, I will show how electrochemical methods
provide complementary information for biofilm characterization. I will
also show ongoing work on electrofermentation.
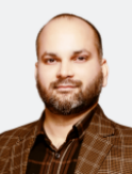
Asst. Prof. Faez Iqbal Khan
Xi'an Jiaotong-Liverpool University, China
Dr. Faez Iqbal Khan is currently serving as an Assistant Professor
within the Department of Biological Sciences at Xi'an Jiaotong-Liverpool
University. He holds a Ph.D. degree in Computational Chemistry
(Bioinformatics) from Durban University of Technology, South Africa. Dr.
Khan has obtained Bachelor's and Master's degrees in Biomedical Science
and Bioinformatics, respectively. Throughout his career, Dr. Khan has
conducted research and teaching across esteemed institutions such as
Rhodes University (South Africa), South China University of Technology,
and the University of Electronic Science and Technology of China. His
main areas of research focus on Protein engineering, Protein folding,
drug design, and Protein dynamics. Dr. Khan established wide-ranging
collaborations with BRICS countries and mentored several postgraduate
students. He has authored over 75 publications in international
peer-reviewed journals, which are well cited.
Speech Title: "Biofilm
Electrochemistry: from Characterization to Applications"
Abstract: This study focuses on
integrating the AI tool AlphaFold with PyMOL for predicting protein
structures and enhancing pedagogical practices in transnational biology
education. Through an action research framework, innovative teaching
strategies are developed to enrich student learning using AI-driven
protein modeling. The study demonstrates the effectiveness of these
methods in improving student motivation, conceptual knowledge, and
digital literacy. Ethical considerations related to the use of molecular
visualization tools in higher education pedagogy are also explored.
Survey participants emphasized the importance of visualization tools
like PyMOL for studying protein structure. This study highlights the
effective design and planning of learning activities by integrating AI
tools and PyMOL, supporting active student engagement with complex
scientific concepts.
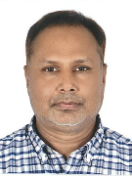
Assoc. Prof. Md. Altaf-Ul-Amin
Nara Institute of Science and Technology, Japan
Md. Altaf-Ul-Amin received B.Sc. degree in Electrical and Electronic
Engineering from Bangladesh University of Engineering and Technology
(BUET), Dhaka, M.Sc. degree in Electrical, Electronic and Systems
Engineering from Universiti Kebangsaan Malaysia (UKM) and PhD degree
from Nara Institute of Science and Technology (NAIST), Japan. He
received the best student paper award in the IEEE 10th Asian Test
Symposium. Also, he received two other best paper awards as a co-author
of journal articles. He previously worked in several universities in
Bangladesh, Malaysia and Japan. Currently he is working as an associate
professor in Computational Systems Biology Lab of NAIST. He is
conducting research on Network Biology, Systems Biology, Cheminformatics
and Biological Databases. He published around 90 peer reviewed papers in
international journals and conference proceedings. Current google
scholar citation index of his publications is more than 8200.
Speech Title: "Novel
Methods and Tool for Clustering of Simple and Bipartite Graphs:
Applications in Ecology and Computational Biomedical Research"
Abstract: Network analysis particularly
graph clustering has become a useful and important technique in data
mining applications. It provides a global view of data structure where
highly concentrated data are grouped based on their common properties.
Previously we developed graph clustering algorithms DPClus and DPClusO.
Recently, we proposed a novel biclustering approach called BiClusO. We
compared our biclustering algorithm with five different algorithms using
biological and synthetic data and evaluated the performances. Our
algorithm shows the best performance over the selected five biclustering
algorithms. We also present new integrated software implementing the
DPClusO and BiClusO algorithms to be utilized for simple and bipartite
graph clustering. This tool provides the user with GUI based facilities
for simple and bipartite graph clustering along with filtering and
amalgamation facilities, hierarchical node analysis, node distribution
among cluster set and visualization of all or partial portion of a big
cluster set. We used this tool to analyze the bipartite relations
between species and volatile organic compounds (VOCs). VOCs emitted by
different species have huge environmental and ecological impacts.
Biosynthesis of VOCs depends on different metabolic pathways based on
which the species can be categorized. Our experiment shows that VOC
based classification is consistent with taxonomy based classification of
the species. Furthermore, we applied simple graph clustering algorithm
DPClusO for finding inflammatory Bowel Disease(IBD) related genes. We
also analyzed the mRNA and miRNA bipartite relations. Finally, we
successfully identified some important IBD related miRNAs. Also, we will
explain KNApSAcK database which has been developed in our lab.
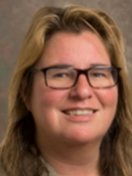
Asst. Prof. Anja Nohe
University of Delaware, USA
Dr. Anja Nohe is an Associate Professor at the University of Delaware,
USA known for her research in cellular signaling and bone biology, with
a special focus on Bone Morphogenetic Protein 2 (BMP2). She has made key
contributions to understanding BMP2’s role in bone formation and
osteoblast differentiation, highlighting its potential in treating
osteoporosis and healing fractures. Her work includes pioneering BMP2
signaling pathways, developing computational models for therapeutic
prediction, and advancing osteoporosis treatments. Additionally, she
explores BMP2 pharmacokinetics for better drug delivery. As a dedicated
mentor and prolific author, Dr. Nohe’s work bridges molecular biology
with clinical applications in bone health.
Speech Title: "Integrative
Computational Modeling of BMP2: Systems Biology and PBPK Insights into
Bone Health and Therapy"
Abstract: Bone Morphogenetic Protein 2
(BMP2) drives critical cellular processes such as differentiation and
tissue homeostasis. Reduced BMP2 expression or activity is associated
with impaired bone formation, decreased bone density, and increased
fracture risk, all of which are characteristic of osteoporosis. BMP2’s
capacity to activate osteoblasts diminishes in these conditions, leading
to an imbalance between bone formation and resorption, which exacerbates
bone fragility. To support a comprehensive understanding of BMP2’s
roles, we created a Physiologically-Based Pharmacokinetic (PBPK) model
to analyze the steady-state distribution of BMP2 in mice. Unique to this
model, BMP2 elimination is driven by receptor kinetics, and BMP2
generation is regulated through protein turnover. We further molecular,
cellular, and tissue-level interactions using a systems biology
approach. This model captures BMP2 signaling pathways, including both
Smad-dependent and Smad-independent mechanisms, to simulate osteoblast
differentiation and bone remodeling. The systems biology approach links
these cellular processes to tissue-level outcomes, helping to clarify
BMP2’s role in maintaining bone homeostasis and supporting therapeutic
development. The PBPK model successfully predicts steady-state BMP2
concentrations in mouse tissues and offers a foundation for scaling
dosage regimens to potential human applications.